Speaker:
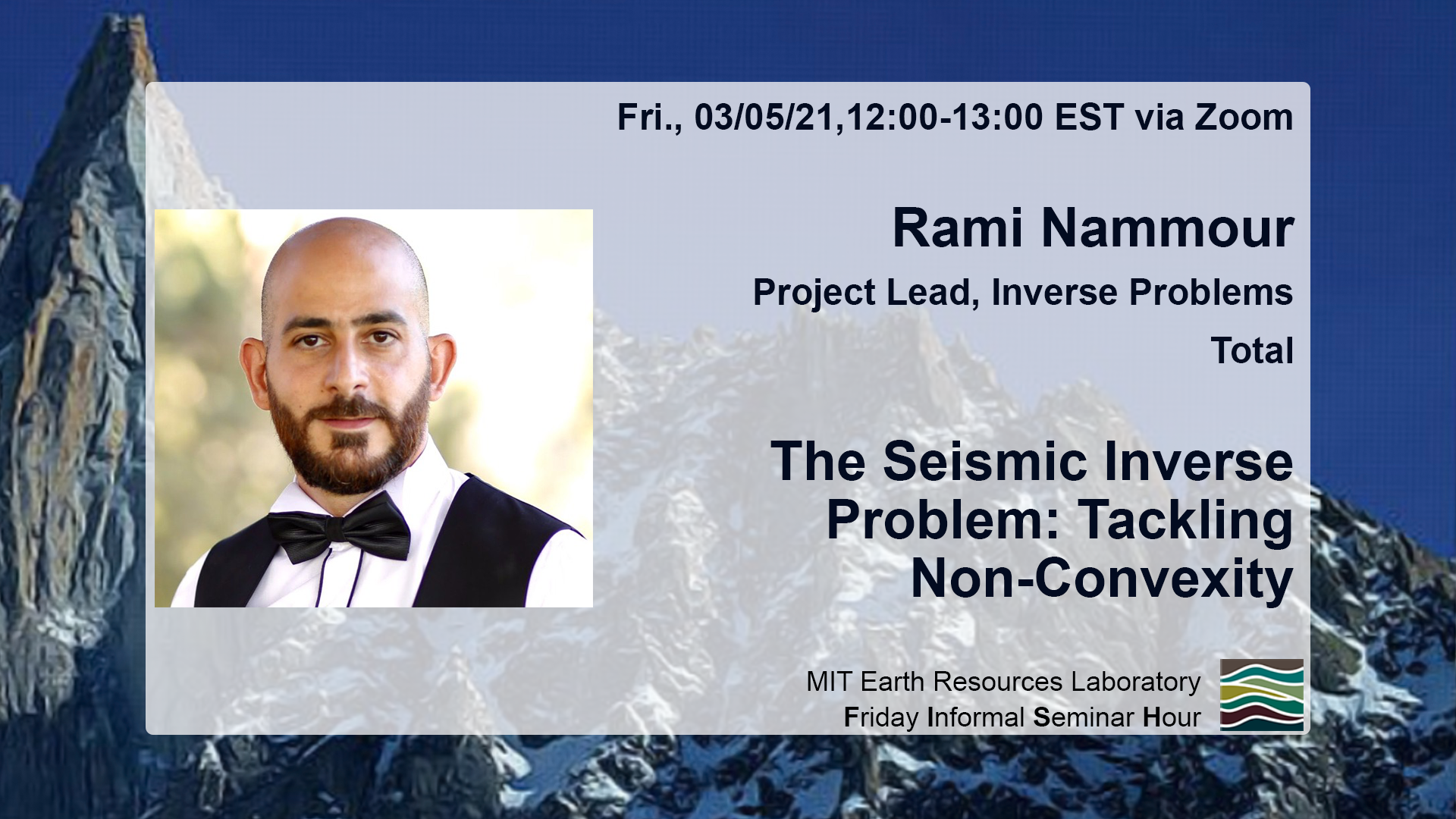
MIT Earth Resources Laboratory presents Dr. Rami Nammour, Project Lead for Inverse Problems and Uncertainty Quantification at Total Research and Technology, on "The Seismic Inverse Problem: Tackling Non-Convexity."
"The inverse problem (IP) of reflection seismology seeks to recover the subsurface velocity from surface measurements of acoustic pressure data. This IP is nonlinear and large scale. Formulating its solution as an output least squares optimization problem (termed FWI: Full Waveform Inversion) leads to a computationally expensive, nonconvex optimization. The large scale of the IP limits feasible solutions to local, gradient based, optimization algorithms. The computational complexity of the IP limits the number of affordable iterations.
This two-part talk proposes two independent methods to deal with the nonconvexity of the IP:
The first proposal relaxes the problem by adding nonphysical degrees of freedom (an extension), fitting the data at any (even incorrect) models, and penalizing the nonphysical degrees of freedom to drive the solution towards the correct model. If successful, the solution of the extended and FWI formulations agree. In this case we relax the uniqueness of the velocity model as a function of source location. This extension is fully nonlinear and can fit all pertinent physics of the problem, we call it Nonlinear Inversion Velocity Analysis. We show how the method is capable of recovering the velocity model in complex cases where FWI fails.
The second proposal numerically investigates the ability of generative modeling (Generative Adversarial Networks and Variational Auto-Encoders) in machine learning to encode the velocity model with a modest number of degrees of freedom. The decimation of the scale of the domain of the IP renders global optimization methods feasible, circumventing nonconvexity. As a first attempt, this study encodes layered 1D media. We show numerically that a learned sparse representation can match the success rate of an explicit sparse representation (available for layered media) for solving the IP."
Dr. Nammour graduated in 2011 with a PhD in Computational And Applied Mathematics from Rice University. He has since been working for Total Research and Technology. He first developed a framework for preconditioning multiparameter inverse problems in reflection seismology as a research geophysicist. As part of his first assignment, he got interested in a new programming language, julia, developed at MIT for technical computing. This prompted his move to a computational science group at Total, where he leads a project on Inverse Problems and Uncertainty Quantification, dealing with various applications from seismic imaging, CO2 sequestration and leak detection for HSE (Health Safety and Environment).